AI Evaluation Frameworks: Toward More Comprehensive Approaches and Promising Global Initiatives
- Roxana Salehi, PhD
- Apr 6
- 5 min read
Updated: Apr 7
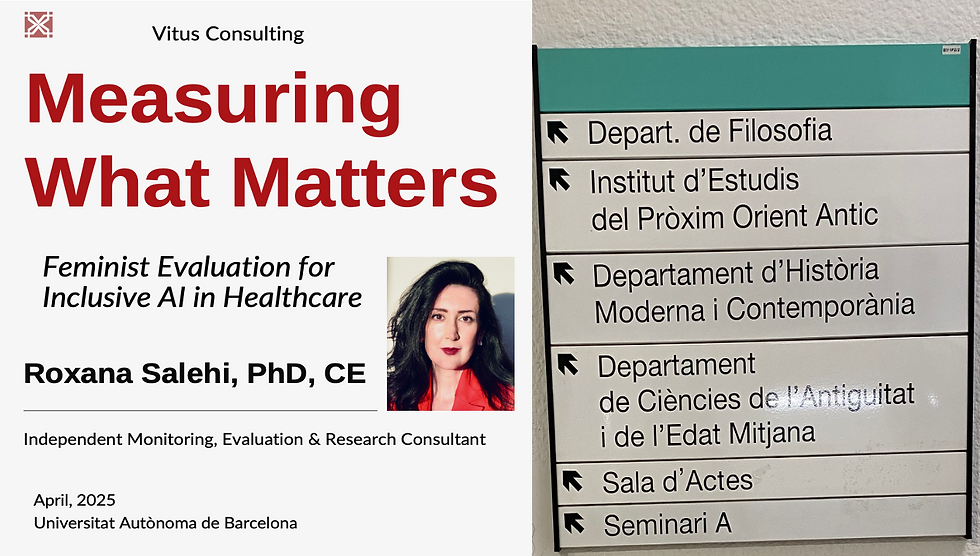
My presentation titled "Measuring What Matters", Department of Philosophy, University Autònoma de Barcelona
I never imagined my first talk in Barcelona would take place in a philosophy department, given my background in health and program evaluation. But that’s exactly what happened, and I’m glad it did. On reflection, it even made sense, given my fondness for good questions and philosophers — oh, they do ask good questions.
The conference, titled “AI, Feminism, and Public Health,” took place on April 2, 2025, at Universitat Autònoma de Barcelona, with Grup d'Estudis Humanístics en Ciència i Tecnologia, organized by Alice Rangel Teixeira. My contribution was to include a program evaluation perspective in this discussion.
In this blog, I focus on one of my key messages: the need for comprehensive AI evaluation frameworks, why comprehensive frameworks are still the exception rather than the norm, and examples of promising initiatives. Let's jump right in:
1. Why Do We Need Comprehensive Frameworks for Evaluating AI?
A thought-provoking moment at the conference came when Paula Petrone, Leader of the Digital Health Unit at Barcelona Supercomputing Center described AI as a mirror, one that helps us better understand ourselves. The audience reacted with questions—some agreed, others pointed out that AI makes mistakes and "hallucinates," while still others disagreed. My own view was that AI simultaneously reflects human ambition to do good and the biases embedded in society — biases that stem from the historical exclusion of marginalized groups. Whichever way you perceive this metaphor, determining what kind of mirror AI could be requires a comprehensive examination of it.
A recent systematic review by Christine Jacob and colleagues published in February 2025 indicated that in the context of healthcare, current evaluation frameworks for AI are lacking in comprehensiveness. They frequently concentrate on technical metrics related to the AI model, while overlooking factors such as clinical impact, integration with clinician’s workflow, or environmental and economic feasibility. This limited focus hinders a complete understanding of AI’s effect on our health and wellbeing and without this understanding, vulnerable populations may be overlooked. In other words, narrow AI evaluation frameworks could hurt health equity.
2. Why Do Most Existing AI Evaluation Frameworks Lack Comprehensiveness?
Many different types of expertise are involved across the AI lifecycle—from development and testing to deployment and post-deployment. Similarly, those evaluating AI come from various fields that have traditionally worked in silos.
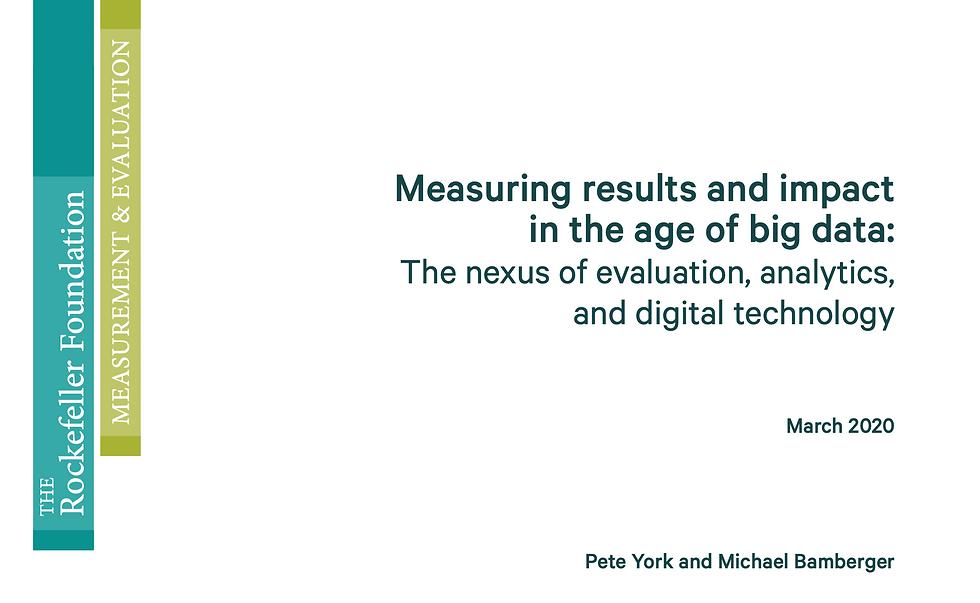
Using data scientists and program evaluators as an example, Peter York, Vice President of Analytic Solutions at BCT Partners, and Michael Bamberger, independent development evaluation consultant, observe that the two groups work in different organizational units, use different tools, attend different conferences, and belong to different professional associations. As I learned in my Machine Learning course with Peter York, the fields also have fundamentally different philosophical underpinnings. One has its roots in frequentist statistics, and the other is Bayesian. One minimizes selection bias but perpetuates the inequities of averages; the other can deliver personalized recommendations but can perpetuate selection biases (details in my previous post).
Recent efforts—through books, special journal issues, and evaluation conference panels—have focused primarily on applying AI tools within program evaluation. But transdisciplinary collaboration to break the silos and co-develop AI evaluation frameworks from the ground up remains rare.
I’m suggesting that everyone involved—computer engineers, data scientists, clinicians, program evaluators, and professionals from other fields—broaden our understanding of evaluation and engage in transdisciplinary work. This means actively integrating insights and methods from different disciplines to create something new that none of the individual fields could achieve alone—ultimately enabling the development of more comprehensive AI evaluation frameworks.
3. The Path Forward: Promising AI Evaluation Frameworks and Global Initiatives
There are some promising efforts emerging with respect to developing comprehensive AI evaluation frameworks. In a previous blog post, I discussed FUTURE.AI Global Guidelines as an example. In the conference I mentioned two other examples: IMPACTS framework and Project AHEAD.
3.1 IMPACTS Framework for AI Evaluation
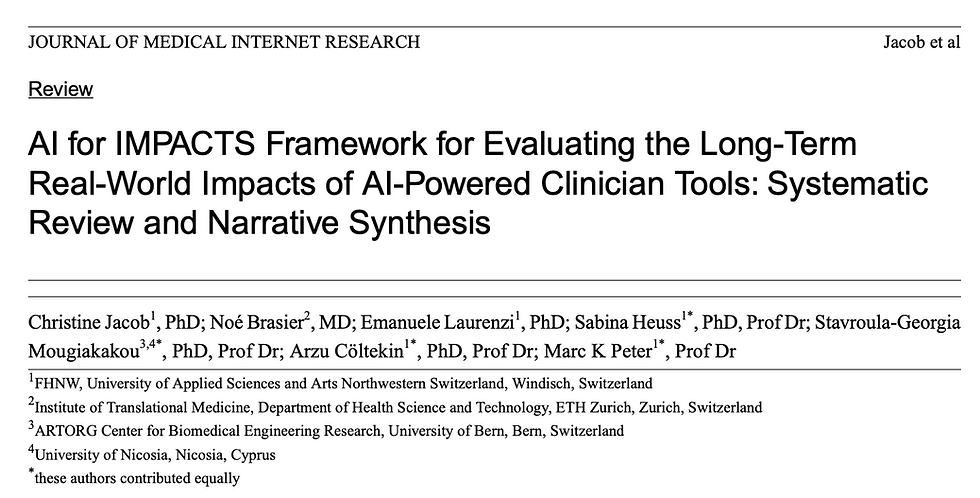
Within IMPACTS framework by Jacob and colleagues, the criteria are organized into seven key clusters, each corresponding to a letter in the acronym:
o I—integration, interoperability, and workflow;
o M—monitoring, governance, and accountability;
o P—performance and quality metrics;
o A—acceptability, trust, and training;
o C—cost and economic evaluation;
o T—technological safety and transparency;
o S—scalability and impact.
You can read the details in their open access article.
3.2 Project AHEAD at Barcelona Supercomputing Centre
AHEAD stands for AI for Health: Evaluation of Applications & Datasets. This project brings together 14 partners from various fields — including medicine and biomedicine, ethics, artificial intelligence (AI) development, gender studies, law, sociology, psychology, and software engineering — with the goal of establishing standards for the responsible implementation of AI in healthcare (Source).
The Barcelona Supercomputing Center is leading the coordination of this European project. Established in 2005, the Barcelona Supercomputing Center specializes in high-performance computing (HPC) and manages MareNostrum, one of the most powerful supercomputers in Europe.
I had the pleasure of meeting some of the super friendly and insightful Project AHEAD team members, thanks to a friend, Julianna Angeova, who sent me a link about the project. Sitting in their high-tech office with beautiful views, I listened as Maria José Rementeria, leader of BSC’s Social Link Analytics team explained the history of this young initiative. Claudia Rosas, and Simona Giardina kindly provide additional contexts about the project's short-term and longer-term objectives.
What really impressed me was their vision for a truly transdisciplinary community. We even talked about anthropology, which was music to my ears. "We aim for people to truly grasp what another field does and learn their approach [to solving problems], engaging in true transdisciplinary work," stated Maria José. And that's the point.
We aim for people to truly grasp what another field does and learn their approach [to solving problems], engaging in true transdisciplinary work. Maria José Rementeria
I am particularly excited to watch where this project goes, as I’m sure it will build bridges with others who are pushing for responsible, trustworthy AI in healthcare — such as the Global Agency for Responsible AI in Health (side note: if you want to join our Community of Practice, you can apply here).
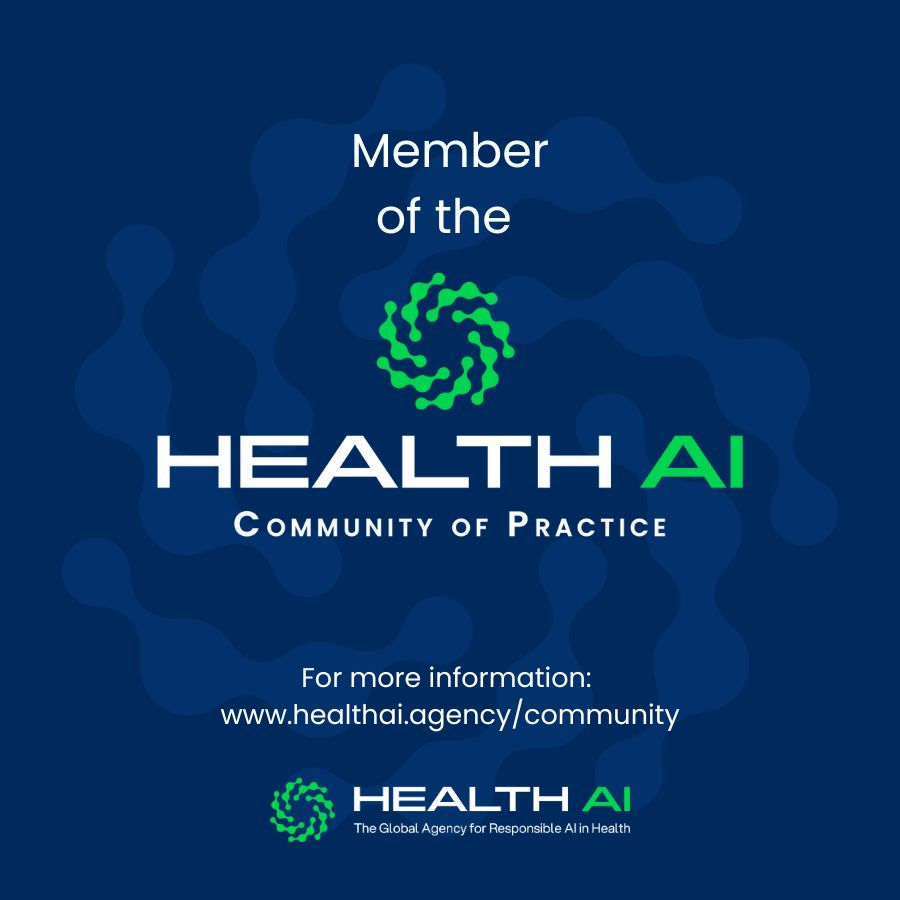
Call to Action
When my dear friend Jesús Martínez heard my talk was held in the philosophy department, he didn't seem surprised and simply said: "We need philosophy more than ever." In this fast-moving world, we need to pause and ask good questions — and asking the right questions about AI evaluation isn't just technical work; it's philosophical, political, and anthropological.
Resources:
Bohni Nielsen, S., Mazzeo Rinaldi, F., & Petersson, G.J. (Eds.). (2024). Artificial Intelligence and Evaluation: Emerging Technologies and Their Implications for Evaluation (1st ed.). Routledge. https://doi.org/10.4324/9781003512493
Jacob C, Brasier N, Laurenzi E, Heuss S, Mougiakakou S, Cöltekin A, Peter M
AI for IMPACTS Framework for Evaluating the Long-Term Real-World Impacts of AI-Powered Clinician Tools: Systematic Review and Narrative Synthesis, J Med Internet Res 2025;27:e67485, URL: https://www.jmir.org/2025/1/e67485
DOI: 10.2196/67485
Lekadir K, Frangi A F, Porras A R, Glocker B, Cintas C, Langlotz C P et al. FUTURE-AI: international consensus guideline for trustworthy and deployable artificial intelligence in healthcare BMJ 2025; 388 :e081554 doi:10.1136/bmj-2024-081554
New Directions for Evaluation: Volume 2023, Issue 178-179
Salehi, R. Unlocking the Potential: Convergence of Evaluation and Data Science, November 2023, available: https://tinyurl.com/255h54pr
Salehi, R. Operationalizing FUTURE-AI Guidelines to Evaluate AI in Healthcare: Where Can Monitoring and Evaluation (M&E) Experts Contribute?, March 2025.
York, P., & Bamberger, M. (2020, March). Measuring results and impact in the age of big data. The Rockefeller Foundation. https://www.rockefellerfoundation.org/wp-content/uploads/Measuring-results-and-impact-in-the-age-of-big-data-by-York-and-Bamberger-March-2020.pdf
The Agency for Health Quality and Assessment of Catalonia (AQuAS), Assessment guide for digital health technologies that use artificial intelligence (AI), November 2024. https://tinyurl.com/r8y4w9bw
Commenti